Capturing the Chaos – How Conversant Grabs Tribal Knowledge
- Manoj Tiwari
- Mar 13
- 4 min read
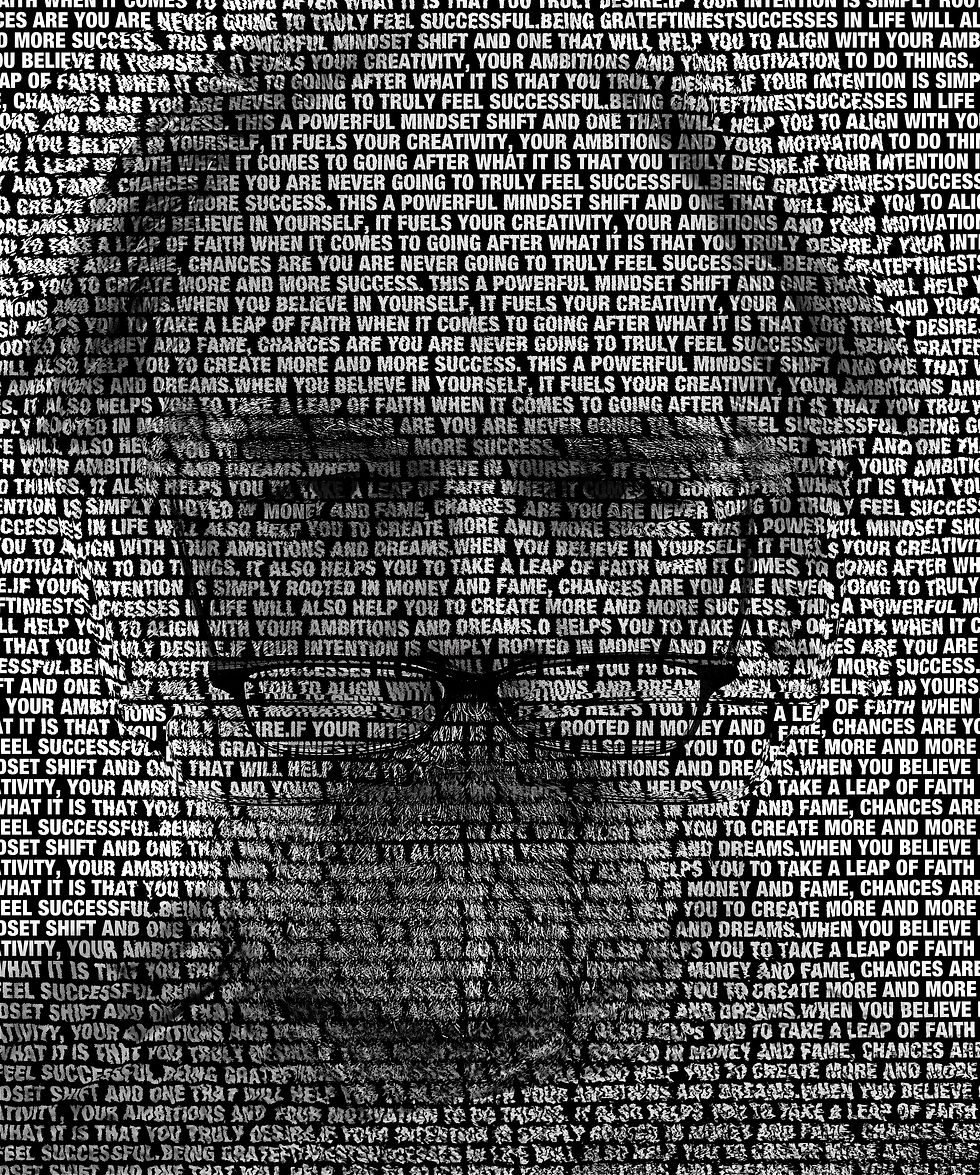
I read over 50 articles, fed hundreds more into AI tools for summaries, and grilled experts—all to crack one question: how do we capture tribal knowledge before it vanishes?
But here’s the real problem—when was the last time you were excited to update a wiki? Probably never. And that hesitation isn’t just an inconvenience; it’s exactly why traditional knowledge management tools fail.
As I dug deeper, I uncovered an uncomfortable truth: half of so-called “tribal knowledge” is outdated, redundant, or outright misleading. But the other half? That’s the gold—the insights companies can’t afford to lose.
Think about that for a second. We’re preserving noise while letting critical expertise slip through the cracks. Yet, hidden within that noise is knowledge that could transform efficiency, training, and decision-making in any industry.
So, how do we separate the gold from the noise?
The Tribal Knowledge Problem: Why Traditional Tools Failed
For over a decade, countless companies have claimed to tackle the tribal knowledge problem. Yet, their solutions almost always boiled down to some form of knowledge management platform, internal wiki, or documentation tool. The intentions were right, but the tools were insufficient.
Ask yourself—when was the last time you were excited to update a wiki or meticulously document a process in some internal system? The reality is, knowledge retention strategies that rely on manual updates inevitably fail because they demand extra effort from already busy experts.
Now, with AI, that’s changing. Experts no longer have to write, update, or maintain documentation manually—AI can work alongside them, interviewing them dynamically, extracting valuable knowledge, and structuring it for usability later. This article is all about how the latest AI techniques can finally crack the tribal knowledge problem in a way that traditional systems never could.
The Three-Step Framework: Identifying, Extracting, and Structuring Knowledge
From my research and our ongoing work at Conversant, I’ve distilled three critical steps:
Identify the right experts – Not all knowledge is equal, and not all experts are the right ones to document it. Finding the true knowledge holders (the ones who solve problems in real time, not just those with seniority) is crucial.
Extract knowledge systematically – A good interview process dynamically adjusts to the expert’s responses, identifying missing links and ensuring knowledge completeness.
Structure it for usability – Raw knowledge capture is worthless if it’s not stored in a format that can be searched, queried, and applied effectively.
In my previous post, Riding the Silver Tsunami – How AI Can Save Your Company’s Tribal Knowledge, I introduced the problem: experts are retiring, and their knowledge is disappearing with them. That post set the stage for this series, outlining the importance of knowledge retention. Now, in Part 1 of this tech series, we focus on the ‘inputs’—how to capture tribal knowledge effectively before it’s lost.
The reality is, if you capture everything indiscriminately, you end up with a bloated, noisy dataset. If you’re too selective, you risk missing the nuances that make expertise valuable. Striking the right balance is both an art and a science.
Hypothesis: 50% of Tribal Knowledge is Waste—But the Other 50% is Priceless
Take, for example, a manufacturing plant where engineers have been following a certain process for years. The tribal knowledge passed down says, “Always preheat the material to 200°F before assembly.” However, no one realizes that this was a workaround for an outdated issue that no longer exists. The new machines actually optimize temperature on the fly, making the preheating step unnecessary. Without proper AI-driven validation, this kind of outdated knowledge persists, leading to inefficiencies and wasted time.
On the flip side, tribal knowledge is priceless when it uncovers hidden optimizations—like an experienced technician knowing that adjusting the feed rate by 5% during winter months prevents defects. AI can help distinguish between these types of insights by systematically verifying, cross-referencing, and dynamically updating knowledge.
This presents a fundamental challenge: how do we sift through the noise to uncover the truly valuable insights?
The Story of Two Companies: Who Gets It Right?
Let me illustrate with two fictional companies facing this exact problem:
Company A took the traditional approach. They recorded hours of interviews, created thousands of pages of documentation, and stored it in a knowledge base. But when employees tried to use it, they found it overwhelming, hard to navigate, and often irrelevant. The project quietly faded into the background, an expensive but unused archive.
Company B, on the other hand, used an adaptive AI-driven approach. Instead of dumping raw transcripts, they built a structured knowledge graph, where insights were dynamically connected, validated, and accessible through intelligent search. Employees could ask real-world questions and get precise, context-aware answers—effectively surfacing only the most relevant 50% of knowledge.
The result? Company B saved time, reduced costly errors, and ensured continuity even when key employees left.
What’s Next: From Tribal Knowledge to Actionable Intelligence
Capturing knowledge is just the beginning. The real value lies in making it actionable—integrating it into workflows, training programs, and decision-making tools.
At Conversant, we’re ensuring that expertise is not only documented but usable, searchable, and continuously refined. The AI doesn't just store data—it validates, refines, and dynamically evolves knowledge over time.
Imagine an AI that doesn’t just capture knowledge but remembers, cross-references, and fills gaps automatically. Instead of experts having to manually update documentation, AI works alongside them—structuring, verifying, and making insights instantly accessible.
That’s the shift we’re making—from static documentation to an evolving, AI-powered knowledge system that keeps expertise alive long after the expert has left the room.
In the next post, Part 2 of this series—Building the Brain – Knowledge Graphs and AI Organization (Processing)—I’ll dive into how AI-driven validation processes ensure that the knowledge we capture remains accurate and useful over time.
Have you experienced the challenge of preserving tribal knowledge?